

With the rapid development of multimedia content-sharing services, there is a growing demand for users to have complete control over audio and video. This trend raises users’ expectations for machine-learning-based audio and video manipulation. This suggests that the relationship between vision and sound can be represented and controlled by machines. This research field is rapidly growing, and many methods for advanced video recognition have been proposed. Most of the recently proposed methods are built on the basis of deep learning. A-V learning has overcome the limitation of recognition tasks with a single modality by using multiple modalities. Focusing on this correlation and the human recognition of sound, researchers have been exploring audio-visual (A-V) learning, such as speech separation, A-V source separation, and A-V self-supervised learning. Based on this correlation, humans can unconsciously combine visual and auditory perception to obtain rich sensory information.

We can recognize sound in these ways because vision and sound have strong correlation and correspondences in the semantic, temporal, and spatial dimensions. For example, a speaker’s appearance enables us to assume the speaker’s voice, the speaker’s lip or body motion indicates the timing of their speech, and the speaker’s position indicates the direction from which their voice is coming when using both ears. This effect is significantly assisted by visual information. Humans can easily recognize and separate each sound, even in a noisy environment, which is known as the “cocktail party effect”.
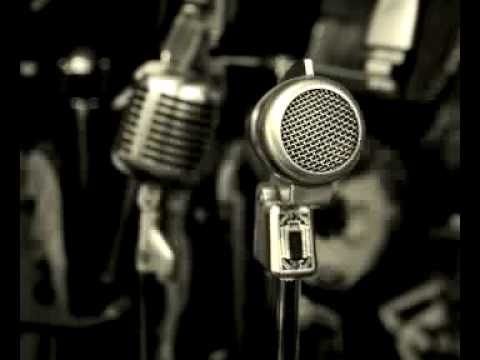
We confirmed the effectiveness of our methods through off-screen sound detection and separation tasks. We conducted our evaluation using generated video data to circumvent the problem of difficulty in collecting ground truth for off-screen sounds. Consequently, the proposed method can separate off-screen sounds irrespective of the direction from which they arrive. Furthermore, we propose a new pre-training method that can consider the off-screen space and use the obtained representation to improve off-screen sound separation. The proposed method separates such off-screen sounds based on their arrival directions using binaural audio, which provides us with three-dimensional sensation. Specifically, sounds coming from outside a screen have no audio-visual correspondences and thus interfere with conventional audio-visual learning. Although such audio manipulation tasks are based on correspondences between audio and video, these correspondences are not always established. In the field of audio-visual analysis, researchers have leveraged visual information for audio manipulation tasks, such as sound source separation. This study proposes a novel off-screen sound separation method based on audio-visual pre-training.
